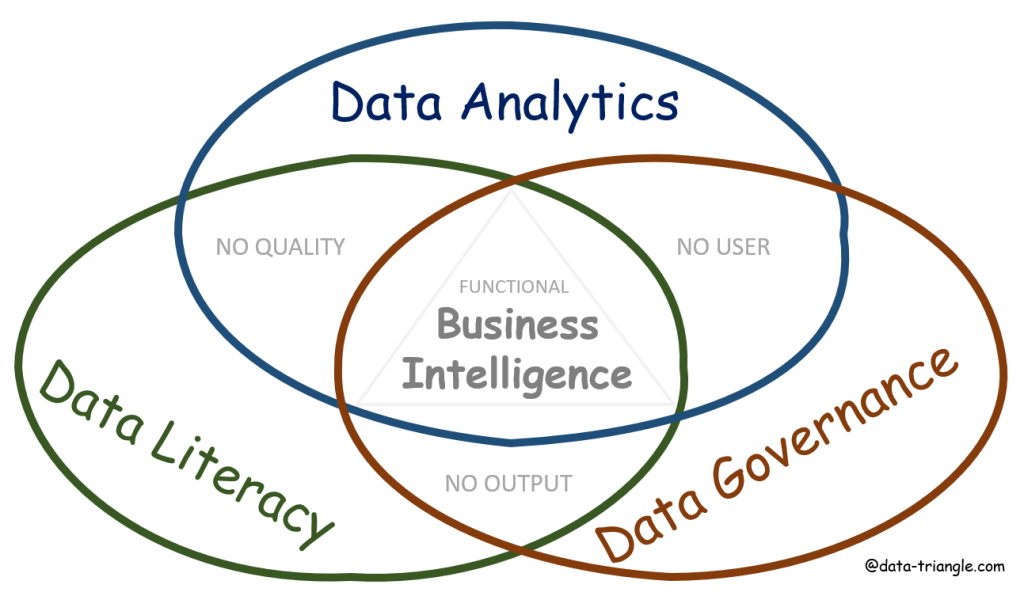
Buzzword or a Real Term
Business Intelligence, Artificial Intelligence, Big Data, Data Science, Advanced Analytics, Dashboarding and many more. These are all terms that we encounter more and more often in the business and not only business environment. However, few of those who use these terms can meaningfully explain them. If we try to find a definition on our own initiative, we get a number of links, each of which explains the concept in its own and often very different way. One may wonder if all the “data” and “intelligence” have any meaning, or if they are just other candidates for corporate “bullshit bingo”. The problem is that the area of data analytics to which these concepts relate is evolving at a rapid pace, and what was true today may not be true tomorrow. The terminology itself is also drawn by marketing purposes rather than technical background.
As a BI analyst, I had to explain these concepts many times to both technical and non-technical people, and it was not always an easy task. In this post, I will try to clarify what the term Business Intelligence means to me. Rather than theory lessons, I will draw on the practice and how I have perceived the field of Business Intelligence over the years.
Data Analytics
Is Business Intelligence just a fancy name for data analytics that has taken hold in a business environment? Data analytics is a wide area involving handling data with a various kind of tools and providing many specific data analysis. Data analysis helps us to understand and benefit from our data. Data analysis in the business environment serves us to understand how our product is perceived, market behavior and other business contexts that should lead to increased company profit. The existence of corporate data that is collected in corporate information systems is essential for data analysis.
Here, I could end my definition by stating that Business Intelligence is a subset of data analytics. In practice, however, I noticed one important thing. Even the best output of data analytics is sometimes received with embarrassment or not used at all.
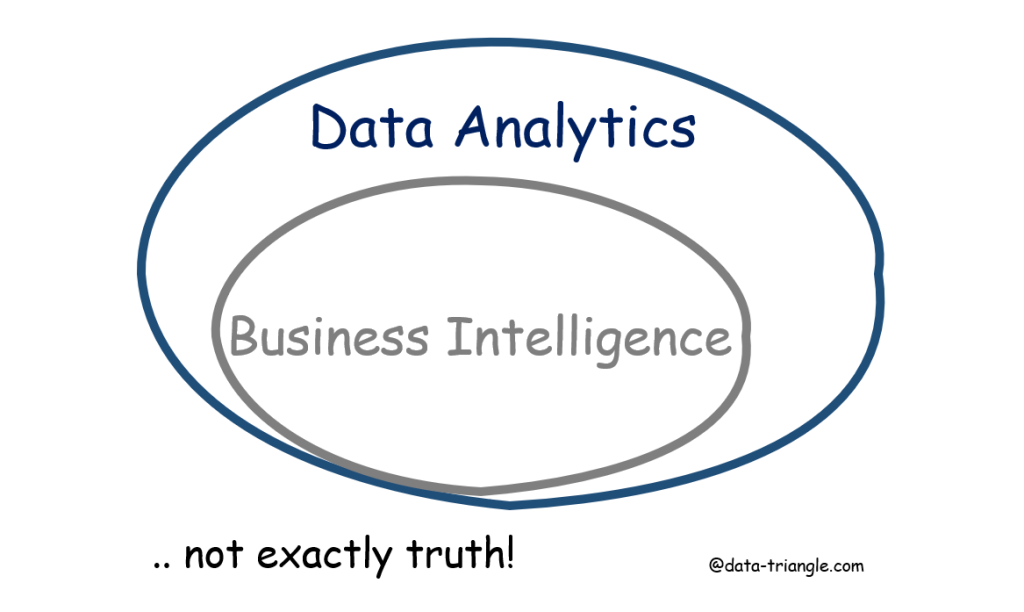
Missing Pieces to the Success
I will give the following example. A data analyst at a large SaaS company was given a task to create an analysis of a customer helpdesk. The task requestor was interested in segmentation according to the industry in which the client operates and how many complaints there are about the website availability. Data analyst identified the data in the source system, verified its sample with the requester and created an interactive dashboard in the BI tool. The dashboard was successful in all respects. It contained filters, industry segmentation based on complete expression and word roots, drill down to ticket detail. Data sample was successfully validated against the source. Shortly after launching the dashboard, however, the analyst began to receive the first complaints. “Company “A” isn’t the pharmaceutical industry, but healthcare, as we have in the CRM system.” “I don’t understand how segmentation was done.” “Where can I find the ticket details?” The data analyst did everything right, rather he did everything correctly regarding the technical task itself.
The problem was that the industry information was entered into the system by the client himself within the ticket, instead of being taken automatically from the CRM system. In the ticketing system, this field did not have a list of allowed values set and was often confused with a job position field below. We received poor quality data already on input. Another reason for the contradictory acceptance of the dashboard lay in the fact that the users of the dashboard were not sufficiently familiar with the functionality of the BI tool.
Why was the dashboard rejected
- The user was not familiar with the functionality of the BI tool
- Poor data quality
I have encountered similar stories, I have been often direct participant, many times during my professional life. From the initial frustration that someone is complaining about a “good” analytics output, it is necessary to move to the point of “what to do to prevent similar situations”.
Even the best analysis is useless if we do not have quality data on input and users who are able to interpret and use the results.
Understanding Data
Data Literacy is becoming an essential skill in today’s world. Anyone who uses a computer for their work should be familiar with the basic concepts associated with data. Being able to read data, interpret it in context and derive further actions based on it. This is true in everyday life, but let’s stick to our business practice purposes. Data literacy is not tied to a specific technical area, it does not require knowledge of programming or a specific tool. It is an understanding of general concepts such as: where data originates from, where it is stored, or what happens to the data before it appears in our dashboard, report or other output.
Data literacy has nothing to do with highly specialized technical skills and needs to be emphasized in both technical and business roles. If both IT and business are aware of the specifics of the data environment, the collaboration will be effective with minimum communication noise. Returning to our example, the “data literate” requester of the dashboard should be aware of where the required data comes from and, if in doubt about its accuracy, contact the administrator of the specific system and clarify the situation. The data analyst should in turn explain to the future user both the functionality of the BI tool and the content of a specific dashboard. Overall, there should be continuous communication between the requester and the dashboard creator. The data analyst himself can process the data well. But only together with the requester, who understands the given areas from a business point of view, they can create a dashboard with added value.
Data Quality
Even the best cooperation between IT and business will not help when we already have bad / poor quality data on input. Quality data is the basis of every analysis and if we do not have it, it makes almost no sense to make additional efforts. However, evaluating data quality in advance, without knowing the broader context, can be difficult. It is necessary to realize that in reality there are only a few datasets that would not suffer from any shortcomings. It is important to be aware of these shortcomings and, if possible, to eliminate them in advance. We will often work with data that is not ideal. At the same time, however, the structure and quality of the data must enable us to achieve the desired goal. Simply illustrated on the example of invoicing – if our system shifts the decimal amount of euro payments by one place to the left, we can still work with the data and create rule to correct this issue. However, if the system randomly assigns the currencies in which the transaction was processed, we cannot do anything without the change in the system settings itself.
BI also has a reverse function, which alerts you of errors in the source systems. If the data analyst performed the technical part well, the errors will come to the light very soon, often in the very beginning of the process. Big help is when the dataset has an assigned owner who is responsible for its quality. As a part of the data governance process, the data analyst can discuss the data directly with the data owner. They should ideally set up measures to prevent such problems in the future.
Business and Data
As can be seen from the lines above, I understand BI as a much broader field than Data Analytics that stands on the border between IT and business. While the data analyst or BI developer is expected to be primarily the expert in the specific technical area, the role of the BI analyst is defined much more broadly. Although the focus of their role is also on data, they should have an overview of the users of the data outputs, as well as the owners of the data, and be in active contact with them. They should combine the roles of a data analyst and a business analyst.
In order to achieve a functional BI environment, it is necessary to combine data analytics with quality data and data literacy of all stakeholders. If we do not have well-established data governance processes, we will end up with reports that the lack of the quality. If we neglect data literacy, our reports will miss the users. I have not yet encountered a case where the company has quality data and high data literacy, and yet they neglect data analytics. But the solution would be very simple. Hire a data analyst – it will be a paradise on earth for them.